News & Articles
Bringing cancer expertise to a doctor near you: Color’s copilot and partnership with OpenAI
Othman Laraki
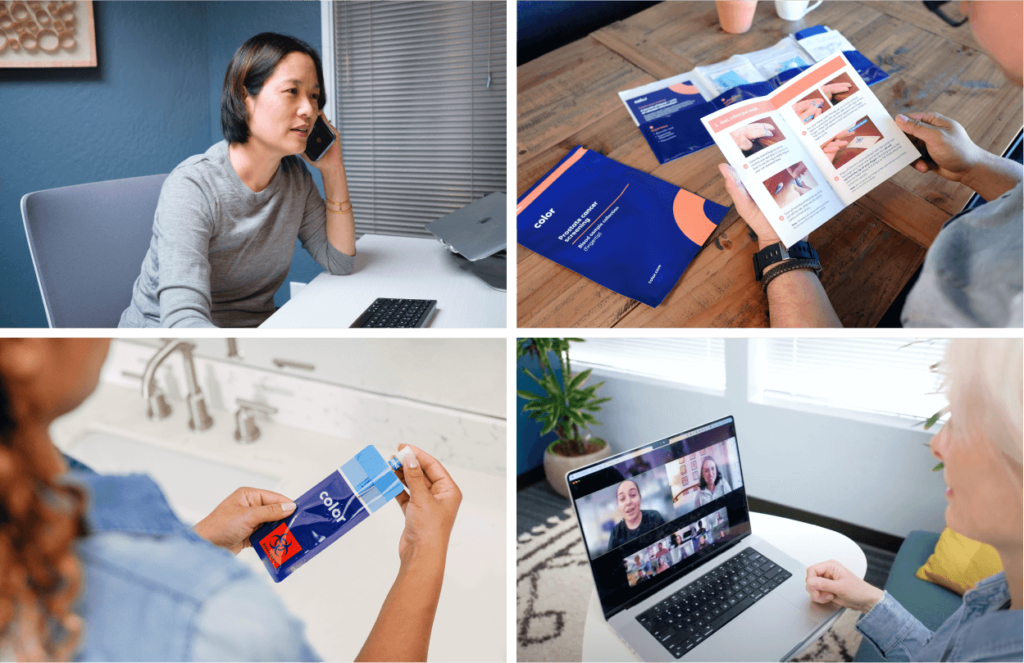
Bringing cancer expertise to a doctor near you: Color’s Copilot and partnership with OpenAI
An idea that has been at the heart of Color’s work since its founding is that technology can improve access and immediacy to critical health information and services. We view the AI revolution we are currently living through as being the single biggest opportunity to deliver on this idea.
Today, we share Color’s copilot, the result of a partnership with OpenAI, where we use GPT-4o to bring the expertise of highly trained oncologists to support doctors, nurses, and primary care physicians in making evidence-based decisions about cancer screening and treatment for their patients. You can read more about the details of our work on OpenAI’s website.
What does the copilot do?
The technology we developed with OpenAI focuses on the two biggest levers for improving cancer outcomes, where access to more immediate, high-quality reasoning can make a big difference.
With new technologies like GenAI, it is tempting to fall into the “to a hammer everything looks like a nail” trap and try to apply it generally to everything. Color’s approach was instead to select a small number of disproportionately impactful use cases in cancer and dive deep to integrate these into clinical workflows. Here is where we’re starting:
1. Catching cancers earlier
The single biggest difference we can make in the cost and outcome of a cancer is to catch it earlier. Not all early-stage cancers are survivable, but across the board, most early stage cancers have a survival rate in excess of 90%.
While there is no silver bullet to catch all cancers early, we have a myriad of screening tools that have been validated and based on which risk-adjusted guidelines have been developed over the years. For example, today, a woman with a BRCA1 mutation which increases her risk of breast cancer can dramatically reduce the odds she will die from cancer by following screening guidelines that are adjusted to her risk level. The challenge arises from the fact that despite the presence of guidelines, the vast majority of women with elevated risk do not receive risk-adjusted guidelines. This happens either because they don’t see a doctor or their doctor does not have the time / background to adjust her screening appropriately.
At Color, we have managed hundreds of thousands such cases – where people can dramatically improve their odds against cancer. Up to now, access to that analysis has relied on a substantial level of expertise which most don’t have access to.
Accordingly, the first use case of Color’s copilot tool focuses on the systems to automate the understanding and analysis of a person’s background risk factors and then apply the guidelines that adjust their screening plan based on their individualized circumstances. We will be starting off by making the output of this work available to the hundreds of thousands of people that are on Color’s Cancer Program – and expect to broaden access to independent primary care doctors after that.
2. Effectively managing patients into treatment
When a person is diagnosed with cancer, their life gets turned upside-down and they often enter a deeply confusing and complex relationship with the healthcare system. For the same reason that an early cancer diagnosis is almost always better than a late stage one, once someone is diagnosed, time is of the essence – where many complex and consequential decisions are made, but need to be made under intense time pressure. Every day counts and every wasted day can impact the outcomes for a patient.
One of the surprising and frustrating steps (both for patients and doctors) is that before a doctor can initiate treatment, they often need to complete what is called a pre-treatment workup. Based on the type of cancer, patient history, and other case specific factors, different tests may be necessary to inform the doctor’s recommendations for treatment. However – in most cases – once someone is referred to an oncologist, she waits for days or weeks for the first appointment, only to be then told that a set of additional tests are necessary to inform the treatment decision. This results in weeks being lost, multiple unproductive appointments for oncologists, and doctors being forced to make treatment decisions with incomplete information to avoid losing even more time.
Our realization is that today, the expertise to decide on pre-treatment workup resides with the oncologist – but access to them requires long waits – long after the diagnosis. So, what we did is build the tooling that brings the expertise to determine what tests are necessary to inform treatment to the primary care doctor’s office or to nurse oncologists. Both of these can jump to action as soon as a patient is diagnosed. That way, by the time the patient meets her oncologist for the first time, she has a much higher chance of being ready to initiate treatment and, we hope, save weeks of precious time.
What is different about this approach?
1. Copilot approach
We decided to take a copilot approach in our work because we believe that the opportunity is less about trying to automate the doctor’s job, but rather by asking ourselves whether we could amplify their opportunity for impact and help them “scale up.” Secondly, we believe that the other side of the “copilot” coin (aside from the AI piece), is to devise user experiences for the users that don’t change their workflows as they exist today. We decided to follow the approach taken with programming copilots, where the successful implementations have been ones that gracefully integrate with engineering workflows as they exist today. Similarly, when thinking about what a copilot could look like for doctors, our belief is that these should not be yet another piece of software that they need to learn and that disrupts their workflow, but rather something that helps them in the background – ideally without even feeling like a new piece of software.
2. Safety & quality orientation
While healthcare applications present some of the greatest opportunities for GenAI, it is also a space where quality and safety are paramount. Beyond the stringent privacy and security requirements imposed by healthcare applications, the high stakes in supporting clinical decision-making by doctors are as high as it gets. One of the most important design decisions behind our work is that the tools were built from the ground up to be based on a human-in-the-loop model. What this means is that in addition to the standard workflow model that clinicians are used to today, our tools were designed around the idea of having a highly available specialist, who just happens to keep getting more efficient over time. To a primary care doctor with a newly diagnosed cancer patient, the Color service offers a fast and efficient service that helps get their patient ready for treatment. This copilot and clinician-in-the-loop pair will just keep getting better/faster over time.
What’s next?
Color is taking a measured approach in rolling out the copilot, using a staged model standard in patient care settings that iteratively evaluates and validates at each implementation phase.
We are starting with an initial phase-in of the copilot with Color Medical physicians applying the tool to a limited number of cases that originate from our cancer management program. These cases receive several layers of quality assurance to ensure that we are performing at a level equal to or better-than what we would get without the tool.
Through the second half of 2024, we anticipate Color’s copilot will have supported over 200,000 patient cases in generating AI personalized care plans, with physician oversight.
If you are a cancer center, oncologist, or healthcare system testing AI applications and want to learn more, please contact us at copilot@color.com.